The growing presence of synthetically created media which digitally alters individuals to appear as someone else, Deepfakes, have been used maliciously to mimic one’s likeness. The ethical topic of Deepfakes runs deep with companies such as Adobe receiving negative publicity for the development of their Deepfake tool Project Morpheus which is being labelled as ‘uncanny’ and ‘manipulative’1. The featured paper in the most recent IEEE Biometrics Council Newsletter (Volume 39 | September 2021), ‘Fighting Fake News: Two Stream Network for Deepfake Detection via Learnable SRM’, puts forward a novel architecture to recognise Deepfakes. The paper proposes the implementation of a two-stream architecture for Deepfake recognition through the incorporation of a steganalysis rich model (SRM) stream and an RGB steam. These said streams would be able to assess the noise information for a given video frame to then determine the likeliness of the video being a Deepfake.
As Deepfakes improve, the technology may pose a risk to biometric recognition as a method to spoof the system in a presentation attack. If the Deepfake is sophisticated enough (left video, sophisticated Tom Cruise Deepfake), or if the biometric identity verification solution lacks the necessary measures against a said presentation attack, the system may be susceptible to Deepfake attacks. The solution to this problem statement proposed within the paper could be implemented within the pipeline of a biometric verification solution to prevent the possibility of a Deepfake spoof. However, the implementation of a two-stream architecture for Deepfake recognition may not be the best solution.
The implementation of the paper’s proposed solution in a biometric identity verification solution would be infeasible as the implementation of the two-stream architecture would create a bloated system that operates slower. Consideration needs to be placed on the ISO/IEC 19795-5 standard, which identifies that high security applications should have an end-to-end transaction duration of less than 6 seconds. The better solution may rely on a biometric system’s techniques to use mechanisms such as texture and patch analysis to combat video screen attacks – which Deepfakes would employ.
In saying that, testing of solutions with presentation attack detection mechanisms in place have been completed in the past by Bixelab through video replay attacks which have great similarity to the Deepfake attacks. That translates to biometric systems informed by the vigorous testing which biometric laboratories such as Bixelab undertake would provide similar resistance against Deepfake presentation attacks. This resistance against Deepfake attacks would be gained after implementing proposed recommendations to increase performance against said presentation attacks.
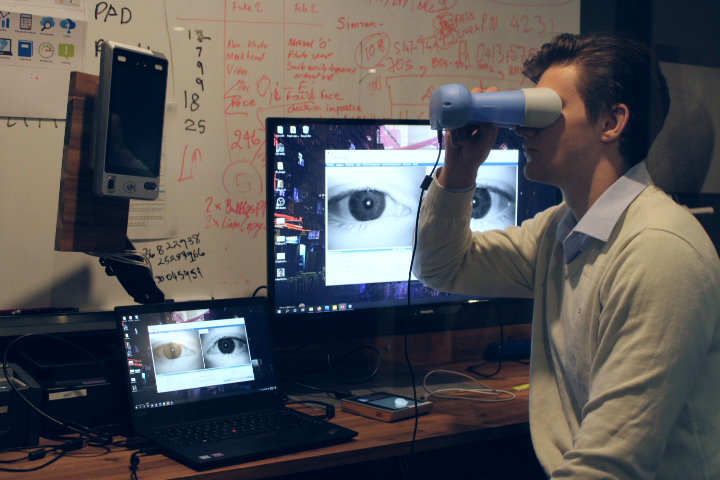
In BixeLab’s general approach, expert testers produce manipulatable deepfakes that are presented with varied screen resolution, video framerate, and screen framerate. This reflects the range of attacker sophistication, utilising latest technologies to attempt to defeat liveness measures of systems tested. With rigorous testing which biometric laboratories such as Bixelab provide, liveness solution owners and sponsors achieve system robustness against current attack vectors.
1James Vincent, “Adobe has built a deepfake tool, but it doesn’t know what to do with it”, The Verge (Oct 27, 2021).